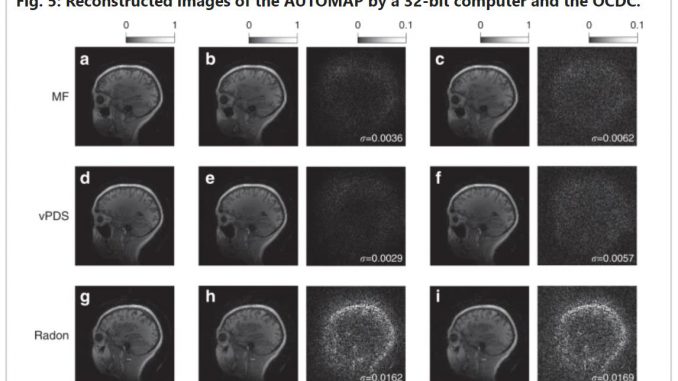
上海交通大学智能微波光波融合创新中心(imLic)博士生徐绍夫的工作——Optical coherent dot-product chip for sophisticated deep learning regression(面向复杂深度学习回归任务的光学点积核芯片)的相关成果近期被Light :Science& Applications期刊接收发表,该工作得到了国家重点研发计划(2019YFB2203700)、国家自然科学基金(61822508)的部分资助。该工作提出一种可以时间复用的光学点积核架构,通过重构可以实现矩阵乘法与卷积的灵活计算。研制出光学点积核芯片,通过片上的精确调控,实现了完整实数域的高精度计算,相对于现有的其他光学计算芯片更能满足复杂深度学习回归任务的需求。利用AUTOMAP模型(现有最佳的图像重构网络模型)执行了高质量的MRI医学图像重构任务,重构的质量接近了32位计算机的理想水平。该工作对光学神经网络领域的进一步发展以及新应用领域具有广泛的借鉴意义。
摘要: Optical implementations of neural networks (ONNs) herald the next-generation high-speed and energy-efficient deep learning computing by harnessing the technical advantages of large bandwidth and high parallelism of optics. However, because of limited hardware scale and incomplete numerical domain, the majority of existing ONNs are merely studied and benchmarked with basic classification tasks. Given that regression (including image reconstruction, gaming, protein-fold prediction, and nanostructure design) is a fundamental form of deep learning and accounts for a large part of current artificial intelligence applications, it is necessary to master deep learning regression for further development and deployment of ONNs. Here, we demonstrate a silicon-based optical coherent dot-product chip (OCDC) capable of completing deep learning regression tasks. The OCDC adopts optical fields rather than intensities to represent values in the complete real-value domain. It conducts matrix multiplications and convolutions in neural networks of any complexity via reconfiguration and reusing, laying the foundation of the sophisticated neural network implementation. Hardware deviations are compensated via in-situ backpropagation control owing to the simplicity of chip architecture, thus enhancing the numerical accuracy of analog computing. Therefore, the OCDC meets the fundamental requirement for regression tasks and we successfully demonstrate a representative neural network, the AUTOMAP (a cutting-edge neural network model for image reconstruction). The quality of reconstructed magnetic resonance images (MRI) by the OCDC and a 32-bit digital computer is comparable. To best of our knowledge, there is no precedent of performing such state-of-the-art regression tasks on ONN chip. It is anticipated that the OCDC can promote novel accomplishment of ONNs in modern AI applications including autonomous driving, natural language processing, medical diagnosis, and scientific study. Moreover, the OCDC and auxiliary electronics have the potential to be monolithically fabricated with CMOS-compatible silicon photonic integration technologies.